
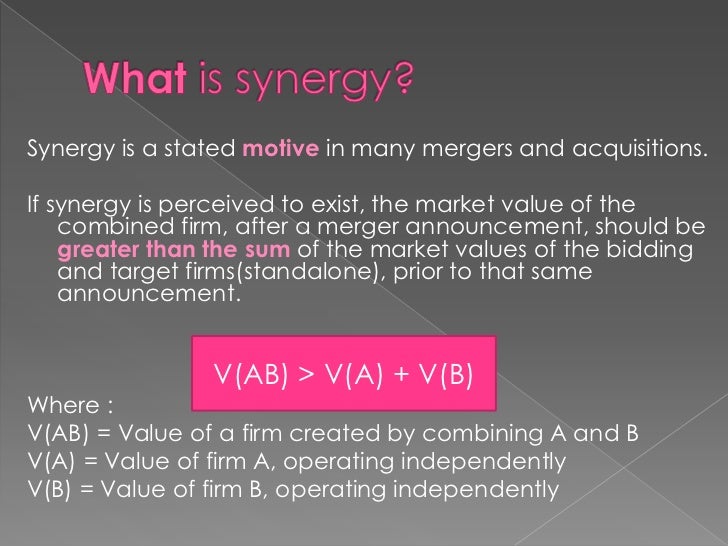
To quickly scan all interactions, a simple measure is needed. From those screens, one needs to filter for interesting candidates that exhibit an interaction effect.

From data generated with high-throughput techniques, one is confronted with massive compound interaction screens. Those interaction effects are usually described as synergistic or antagonistic, dependent on whether the interaction is positive, resulting in greater effects than expected, or negative, resulting in smaller effects than expected. When combining a substance with other substances, one is generally interested in interaction effects. We show this on two datasets of compound combinations that are categorized into synergistic, non-interactive, and antagonistic. Further, we show that computing synergy as lack-of-fit outperforms a parametric approach. In the current study we show that this Explicit Mean Equation outperforms the original implicit formulation of Loewe Additivity and Bliss Independence when measuring synergy in terms of the deviance between measured and expected response, called the lack-of-fit. In a previous study, we introduced, an explicit formulation of the hitherto implicitly defined Loewe Additivity, the so-called Explicit Mean Equation. A non-interactive response is based on a principle of no interaction, such as Loewe Additivity or Bliss Independence. Those promising candidates are chosen based on the deviance between a measured response and an expected non-interactive response. With the help of high-throughput techniques, a huge amount of compound combinations can be screened and filtered for suitable candidates for a more detailed analysis. In synergy studies, one focuses on compound combinations that promise a synergistic or antagonistic effect. 3Center for Integrative Neuroscience, University Tübingen, Tübingen, Germany.2Max Planck Institute for Developmental Biology, Tübingen, Germany.
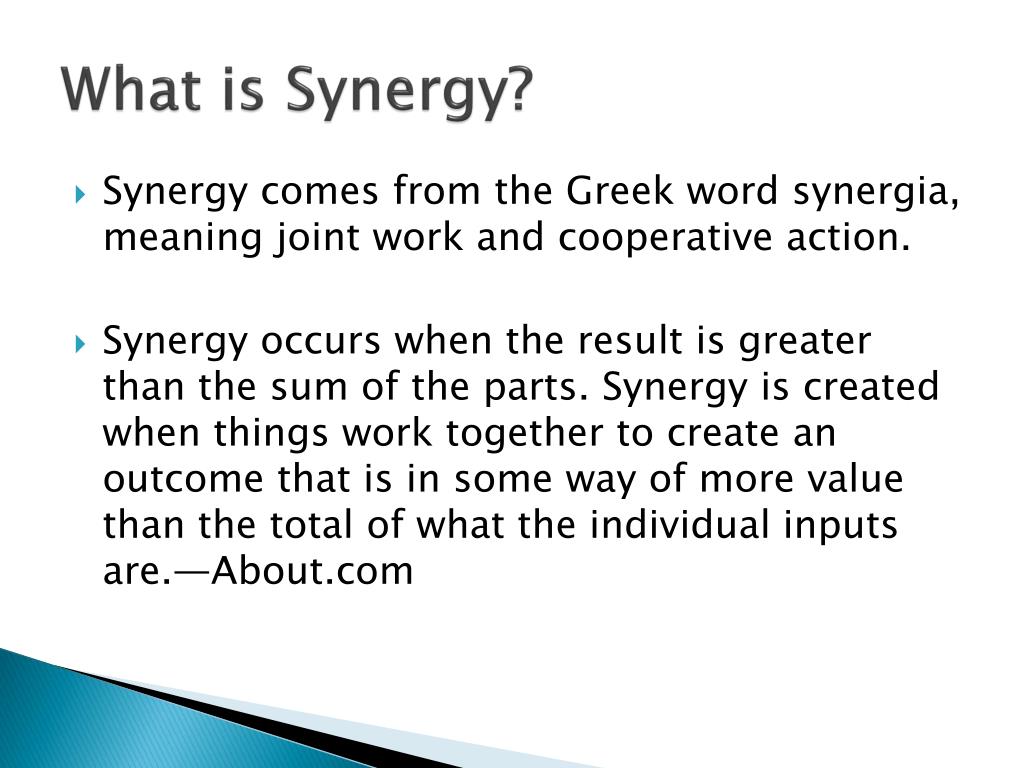
